Four New Center for Nursing Research Pilot Projects Awarded
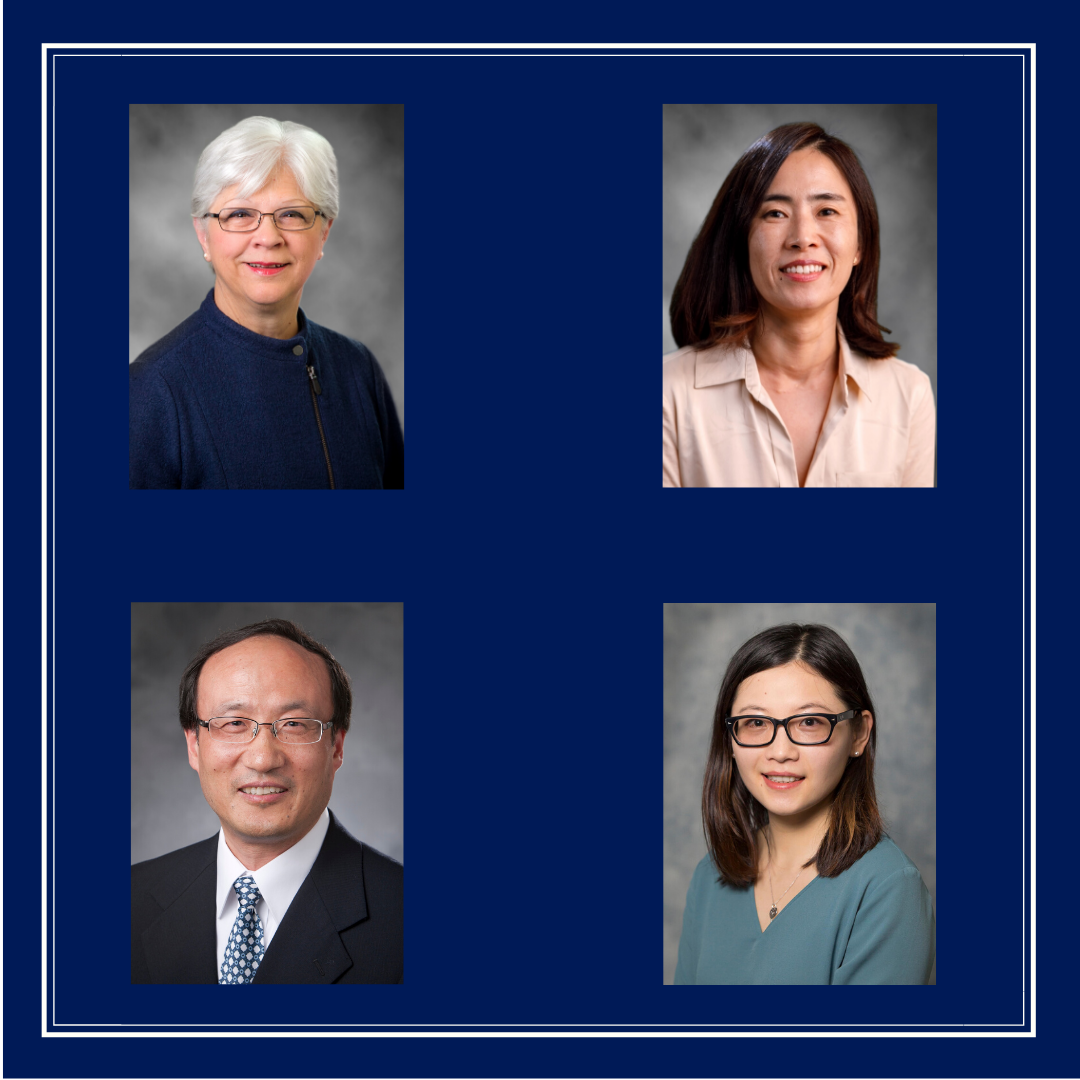
Four teams of researchers and their collaborators have been awarded new funding for pilot projects beginning this spring. Awardees include Associate Professors Debra Brandon, PhD, RN, CCNS, FAAN; Hyeoneui Kim, PhD, MPH, RN, FAAN; Wei Pan, PhD; and Medical Instructor Hanzhang Xu, PhD.
The Center for Nursing Research (CNR) Pilot Program encourages researchers to explore novel, current and innovative ideas that address the School’s Research Areas of Excellence (RAEs). The four RAEs are: data science, health innovation, population health and precision health. The goal of the Program is to encourage team science, and promote scientific inquiry that positions investigators to be competitive for extramural research funding. The Program is administered by the CNR and funded through a generous award from the Chancellor. The pilot program has funded 21 projects since 2017.
For her study entitled Predictive Factors in the Development of Skin Health in Preterm Infants, Principal Investigator Brandon will collaborate with Noelle Younge, MD, assistant professor of pediatrics, division of neonatology. Their study addresses how routine intrusive nurse caregiving activities – like diaper change frequency – can be modified to minimize infant stress without compromising the skin health of very low birthweight preterm infants (VLBW). Preliminary data supports the fact that a diaper change negatively affects physiologic stability. This study will use video data to document the links between caregiving activities and physiological instability (e.g. heart rate, respiratory rate and oxygen saturation). In addition, the team will merge diapered skin health data collected on the skin and gut microbiome, skin pH, and skin trans-epidermal water loss (TEWL) with new stool metabolomic analyses to understand the relationship between metabolites in stool and the microbiome of the diaper-area of the skin. Together, this information will allow Brandon and her team to develop personalized interventions that minimize infant stress while maximizing skin health.
Principal Investigator Kim will team up with Duke University Heath System’s Director of Nursing Research and Evidence-Based Practice Deborah “Hutch” Allen, PhD, RN, CNS, FNP-BC, AONP; the Director of Research at Duke Integrative Medicine, Oliver Glass, PhD; and Assistant Professor of Nursing, Qing Yang, PhD. The project addresses Promoting Physical Activity in Cancer Patients Undergoing Treatment with Personalized Exercise Planning and Tracking. Exercise has been shown to aid in prevention, and improve therapeutic response, adverse effects, psychological well-being and general quality of life in cancer patients, yet only 10% of patients undergoing treatment adhere to exercise recommendations. Cancer experiences and exercise regimens are not “one size fits all,” thus Kim and her team hope to develop an exercise planning and tracking (EPT) tool that personalizes exercise plans to patients’ preferences and constraints. The EPT tool builds on Kim’s previous work developing Physical Activity Ontology (PACO). PACO describes concepts and categories for physical activity as well as how they relate to each other. The EPT tool will consist of three layers: a data layer, an application layer and a web-based user-interface layer that work together to customize an individual’s exercise program. The tool will be pilot tested in a small sample of newly diagnosed cancer patients, and assessed for physical activity readiness, level, functional capacity, cancer-related symptoms and satisfaction with the program.
Principal Investigator Pan and Co-Investigator Bryce Reeve, PhD, professor in population health sciences and director of the Center for Health Measurement, will tackle the issue of symptom clusters in patients with chronic conditions in their project entitled Exploring Machine Learning Techniques for Biclustering Heterogeneous Patient Population with Multidimensional Symptoms. Why is that young children with leukemia may experience clustering of severe fatigue and depression but adolescents with leukemia may experience clustering of severe nausea and sleep disturbances? Biclustering is a machine learning approach developed for the study of gene expression data; it allows for the simultaneous clustering of columns and rows to be structured like a matrix. Pan and Reeve aim to modify the technique for use in evaluating symptom data, where the rows contain patient data and the columns contain symptom data. Such simultaneous analysis could reveal clinically meaningful information about which subgroups of patients experience similar patterns of concurrently identified specific symptom clusters. Once the researchers evaluate the biclustering algorithms in a simulation setting, the next step is to apply the new methodology to real-world symptom data from the Surveillance, Epidemiology, and Ends Results (SEER) cancer registries. If successful, the results will lead to the development of triclustering techniques, in which time – as a 3rd dimension can be added to the model.
Black and Hispanic older adults are much more likely to be diagnosed with Alzheimer’s disease and Related Dementias (ADRD) at later stages than Whites, despite having greater risk factors for ADRD and a higher prevalence of the disease. To address this disparity, Principal Investigator, Xu, along with Kim, Associate Professor in Population Health Sciences, Matthew Dupre, PhD, and Neurology Professor, James Burke, MD, will explore Racial and Ethnic Differences in Care Pathways to Diagnosis of Alzheimer’s disease and Related Dementias. Because the diagnosis of ADRD is complex and involves multiple providers, procedures, and healthcare settings, the pathways to ADRD diagnosis can vary significantly across patients. Therefore, there is an urgent need to identify the distinct pathways that lead to ADRD diagnoses, understand how these pathways differ among racial/ethnic groups, and determine which pathways will promote early diagnosis. This project will draw from life course theory, a theoretical framework that integrates timing, duration and sequencing of care. The overall objective is to leverage routinely collected EHR data and utilize advanced sequence analysis techniques to develop actionable knowledge that will significantly advance clinical practice and promote the early diagnosis of ADRD among diverse older adults.